The ROI of AI
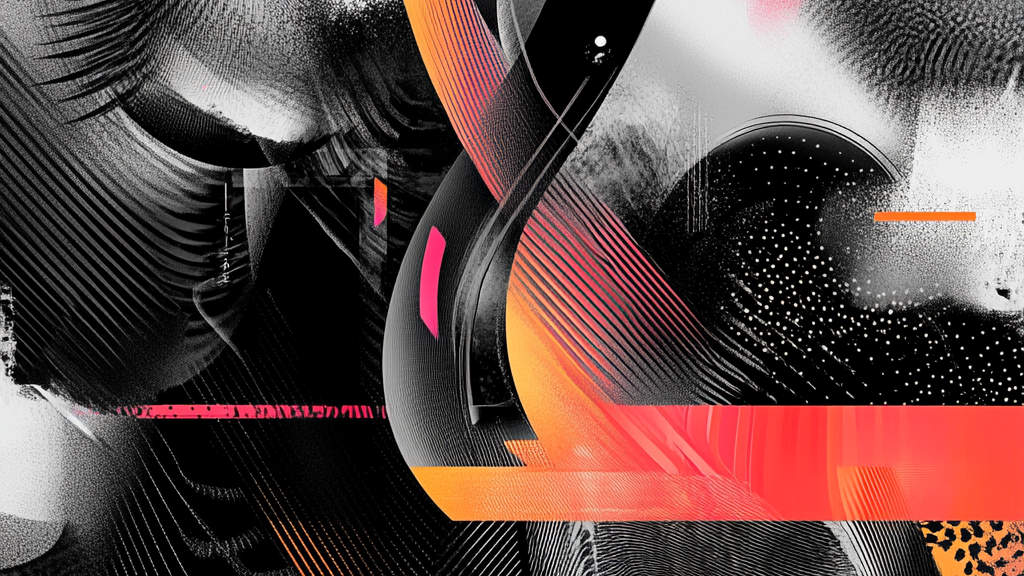
Building A Case for Executive Buy-In
The AI Investment Question
CMOs today face mounting pressure to justify AI investments. The promise of AI in marketing is well understood—greater efficiency, smarter decision-making, and improved performance. Yet, when it comes time to prove AI’s financial return, many marketing leaders struggle to make the case.
Executives don’t invest in AI because it’s innovative. They invest because it creates measurable business value. Without a clear financial argument, AI remains an experimental tool rather than a core driver of growth.
The problem isn’t that AI lacks potential—it’s that too many AI projects are launched without a defined ROI model.
A marketing leader rolls out AI-driven predictive analytics to improve campaign efficiency. The tool enhances audience segmentation and adjusts media spend dynamically, yet budget approvals remain static, delaying AI-driven optimizations. The result? The expected cost savings never materialize.
Another CMO invests in AI-powered personalization to boost engagement and sales. AI-generated recommendations increase click-through rates, but sales cycles remain unchanged because customer interactions are still managed manually. Despite AI improving marketing metrics, revenue impact is negligible.
The issue in both cases isn’t whether AI works—it’s that the financial value wasn’t quantified upfront.
For AI to secure executive buy-in and budget, CMOs must build a business case grounded in revenue impact, cost savings, and efficiency gains. That means moving beyond AI hype and making the financial argument that AI is not just an operational upgrade—but a profit-generating asset.
This chapter will break down how to:
- Define the business problem AI solves and its financial impact.
- Identify and quantify revenue-generating AI use cases.
- Calculate AI’s contribution to cost savings and efficiency gains.
- Build a financial model to measure and predict AI’s ROI.
- Present a compelling case for AI investment at the executive level.
Marketing budgets are scrutinized more than ever. The CMOs who can’t quantify AI’s ROI won’t secure funding for AI initiatives. Those who can will gain a competitive edge—not just in technology adoption, but in marketing leadership.
Why CMOs Struggle to Prove AI’s ROI
AI adoption in marketing has accelerated, but proving its financial impact remains one of the biggest challenges for CMOs. Despite AI’s potential to drive efficiency, precision, and growth, many marketing leaders face resistance from executive teams when it comes to securing budget for AI initiatives.
The issue isn’t whether AI can improve marketing performance—it’s that too many AI investments lack a clear financial model. Without a defined ROI framework, AI remains an operational experiment rather than a strategic business driver.
The Common Pitfalls in AI ROI Justification
Before diving into how to calculate AI’s financial return, it’s important to understand why CMOs often struggle to prove AI’s value. The most common reasons include:
1. AI Adoption is Too Fragmented
Many AI investments start as isolated tools—an AI-powered chatbot here, an AI-driven ad optimization platform there. While each tool may show incremental improvements in specific areas, they aren’t integrated into a unified marketing strategy.
Executives don’t evaluate AI tools in silos—they assess the overall business impact. If AI investments aren’t connected to broader revenue and efficiency goals, they won’t appear on the radar of CFOs or CEOs as strategic priorities.
Example: A marketing team implements AI-driven content optimization, improving engagement rates by 20%. But because lead conversion rates remain unchanged, AI’s impact on revenue is unclear.
2. AI ROI Isn’t Defined Upfront
Many CMOs invest in AI expecting general improvements in efficiency but fail to define specific financial outcomes before implementation.
AI-driven personalization may increase customer engagement, but does it actually increase customer lifetime value (LTV)? AI-powered audience segmentation may improve click-through rates, but does it translate into higher sales?
Without clear, quantifiable success metrics, AI remains a “nice to have” rather than a core driver of revenue growth.
Example: A CMO implements AI-powered lead scoring. The tool predicts high-intent prospects, but sales teams don’t adjust their approach based on AI insights. The result? No measurable impact on revenue, leading executives to question the investment.
3. AI Investments Focus on Cost Savings Over Revenue Growth
A common mistake in AI business cases is focusing solely on automation and efficiency gains. AI-powered automation may reduce marketing execution time by 30%, but unless that translates into lower costs or higher output, it doesn’t move the business forward.
Executives prioritize investments that drive top-line growth, not just operational efficiency. AI’s role in increasing revenue—through better targeting, higher conversions, and smarter customer engagement—must be at the center of the business case.
Example: A company invests in AI-driven media buying to optimize ad spend, reducing cost per lead (CPL) by 25%. But if total revenue from those leads doesn’t increase, the CFO sees AI as a cost-cutting tool, not a revenue driver.
4. CMOs Struggle to Connect AI Improvements to Financial Outcomes
Many marketing KPIs—engagement rates, time on site, customer sentiment—don’t directly translate to revenue. While AI may improve these metrics, the CFO doesn’t sign off on AI budgets based on engagement rates alone.
The challenge for CMOs is bridging the gap between marketing performance improvements and tangible financial impact. This requires aligning AI metrics with business-level outcomes such as:
- Higher revenue per customer
- Lower customer acquisition costs (CAC)
- Increased marketing ROI
- Shorter sales cycles
If AI-driven improvements can’t be connected to profitability, efficiency, or revenue growth, they won’t be seen as mission-critical investments.
Example: AI-powered churn prediction identifies customers likely to leave. The marketing team launches a retention campaign based on AI insights, but if customer retention doesn’t improve measurably, the impact of AI remains unproven.
The AI Business Case Formula: Connecting AI to Financial Impact
Marketing leaders don’t struggle with understanding AI’s potential—they struggle with proving its financial return. It’s one thing to know AI can improve efficiency, optimize campaigns, and personalize customer interactions. It’s another to connect those improvements to revenue, cost savings, and business growth in a way that convinces the CFO and CEO that AI is a sound investment.
Executives don’t approve budgets for AI because it’s cutting-edge—they approve it because it delivers measurable impact to the bottom line. That means CMOs must go beyond broad claims about AI’s benefits and get specific: How will AI generate revenue? Where will it reduce costs? How does it improve marketing execution in ways that lead to profit?
To answer these questions, CMOs need a structured way to measure AI’s return on investment.
How AI Actually Creates ROI
AI delivers financial returns in three fundamental ways: increasing revenue, reducing costs, and improving efficiency. Every AI use case in marketing—whether it's predictive analytics, automated media buying, or AI-powered personalization—must be tied back to one of these core value drivers.
1. AI as a Revenue Driver
AI’s impact on revenue is most visible in how it enhances customer acquisition, conversion, and retention. Marketers have long struggled with the unpredictability of customer behavior, but AI changes that.
Consider AI-powered personalization. Instead of relying on broad audience segments and static messaging, AI dynamically adapts content, product recommendations, and email sequences in real time. The result? Customers engage more, buy more, and return more often. A company implementing AI-driven personalization could see conversion rates increase by 12%, directly translating to millions in additional revenue.
In B2B sales, AI-driven lead scoring ensures sales teams focus on high-intent prospects rather than chasing cold leads. If AI increases the win rate on marketing-generated leads by 10%, that could mean an additional $5M in closed deals annually.
AI also accelerates sales cycles, enabling marketing to nurture prospects more effectively and identify purchase signals earlier. What once took weeks of manual lead qualification can now happen instantly, reducing time-to-close by 15% and unlocking faster revenue generation.
2. AI as a Cost-Saving Tool
While revenue growth is the most compelling argument for AI, cost efficiency is often the first area where AI delivers immediate impact.
Take AI-driven media buying. Instead of relying on manual bid adjustments and gut-feel budget allocations, AI continuously reallocates spend based on real-time performance data. This eliminates wasted ad spend and ensures every dollar works harder. If AI optimizes media performance by just 20%, that can mean millions in reduced acquisition costs.
AI also removes inefficiencies in execution. Tasks that once required large teams and long hours—such as reporting, campaign optimization, and customer segmentation—are now automated. If AI-powered automation saves a marketing team 2,000 hours per year and reduces the need for additional headcount, that translates into hundreds of thousands of dollars in labor savings.
Beyond advertising and labor costs, AI reduces churn-related expenses. Retaining an existing customer is 5x cheaper than acquiring a new one, and AI-driven retention programs can proactively prevent churn, increasing customer lifetime value (LTV) and lowering revenue leakage.
3. AI as an Efficiency Multiplier
Marketing is often slowed down by static processes, slow approvals, and reactive decision-making. AI changes the speed of execution, making marketing not only more effective, but faster and more adaptable.
Instead of waiting for campaign results to make adjustments, AI analyzes data in real time, identifying what’s working and reallocating resources immediately. Creative performance, audience segmentation, and bidding strategies are adjusted continuously, removing the delays that traditionally plague marketing execution.
Consider a brand running A/B tests on ad creative. Traditionally, results would be analyzed after a few weeks, and adjustments would be made manually. With AI, the system can recognize winning variations in hours, not weeks, automatically scaling what works and shutting down underperforming ads in real time.
At scale, this kind of instant optimization means higher-performing campaigns, lower operational friction, and more agile marketing execution.
The ROI Formula for AI in Marketing
To quantify ROI, CMOs should use this framework:

Where:
- Revenue Gains = Additional revenue generated from AI-driven improvements.
- Cost Savings = Reduction in labor, advertising, or operational expenses due to AI automation.
- AI Investment = Software costs, implementation fees, training, and ongoing maintenance.
While the formula itself is straightforward, the real challenge is ensuring that AI’s contributions to revenue and cost savings are accurately measured.
Applying the Formula: AI ROI in Action
A marketing team invests $600K in AI-powered automation, implementing predictive analytics, content generation, and automated media buying.
After implementation, the results include:
- AI-driven personalization increases conversion rates by 10%, leading to $3M in additional revenue.
- AI-powered ad optimization reduces cost per acquisition by 15%, saving $500K in marketing spend.
Now, let’s apply the ROI formula:
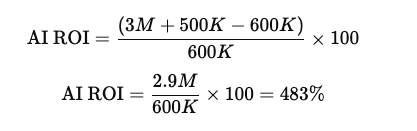
In this scenario, the AI investment delivers a 483% return—a clear and compelling argument for AI’s financial impact.
Step-by-Step Guide to Calculating AI ROI in Marketing
AI adoption in marketing should never be an experiment—it should be a measurable investment with a clear financial return. Yet, many CMOs struggle to quantify AI’s impact in a way that resonates with executives.
To make the case for AI, CMOs need more than promises of efficiency and automation. They need a structured approach to calculating ROI—one that connects AI to tangible revenue gains, cost savings, and operational efficiencies.
This section breaks down a five-step framework for measuring AI’s financial return, ensuring AI investments are strategic, justifiable, and scalable.
Step 1: Define the Business Problem AI Solves
AI is not a solution in search of a problem—it should be applied strategically to address a specific business challenge. The first step in calculating ROI is to identify where AI can move the needle.
Start by asking:
- What marketing challenge is AI solving?
- What is the financial impact of this problem?
- How will AI improve this metric in a measurable way?
For example, if customer churn is a major issue, AI-powered personalization can improve retention by delivering hyper-relevant messaging and experiences at scale. If conversion rates are stagnating, AI can refine targeting, optimize ad spend, and improve lead quality to increase deal velocity.
Calculating the Financial Impact
Consider a company that loses $5M annually due to customer churn. If AI-powered personalization reduces churn by 15%, the projected revenue gain is:
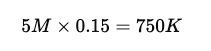
This means that, if AI is successful in achieving this improvement, it will generate $750K in annual revenue retention.
Before moving forward, CMOs must ensure that every AI investment starts with a clear financial problem and a quantifiable improvement target.
Step 2: Identify Revenue-Generating AI Use Cases
Once the problem is defined, the next step is identifying which AI-driven strategies can directly increase revenue. AI’s value lies in its ability to enhance customer acquisition, optimize marketing performance, and accelerate sales cycles.
Common revenue-generating AI use cases include:
- AI-powered personalization: Higher engagement, improved conversion rates.
- Predictive lead scoring: Sales teams prioritize high-intent prospects.
- AI-driven campaign optimization: Smarter media allocation, increased ad ROI.
- AI-powered product recommendations: Higher average order value (AOV) and repeat purchases.
Applying the Formula to Revenue Growth
A company generates $20M in annual revenue from digital sales. AI-driven personalization improves conversion rates by 10%, leading to:
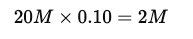
This translates to a $2M revenue increase directly attributed to AI.
The key is to ensure that AI’s revenue contribution is tied to real performance improvements, not just speculative benefits.
Step 3: Quantify Cost Savings from AI
Beyond revenue growth, AI creates measurable cost savings by automating manual tasks, improving ad spend efficiency, and reducing operational overhead.
AI reduces costs in three key ways:
- Automation: AI eliminates repetitive tasks, reducing the need for additional headcount.
- Ad spend optimization: AI adjusts budgets in real time, eliminating wasted spend.
- Operational efficiency: AI-driven workflows reduce marketing execution delays.
Applying the Formula to Cost Savings
If AI automation eliminates the need for three full-time roles at $100K each, that’s a $300K reduction in labor costs.
If AI-driven media optimization reduces wasted ad spend by 20% on a $5M budget, that results in a $1M savings.
Total cost savings:
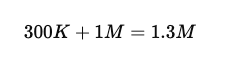
AI’s ability to improve efficiency and cut costs is a major factor in justifying investment, but these savings must be clearly defined and linked to measurable marketing outcomes.
Step 4: Calculate the Full AI Investment Cost
No AI initiative is free, and ROI calculations must account for the total cost of ownership. AI investments extend beyond software—CMOs must factor in implementation, training, and ongoing maintenance.
Key AI cost considerations:
- Technology costs: AI software licensing, implementation, and integration.
- Training & adoption costs: Employee training and process adjustments.
- Ongoing maintenance: AI requires continuous monitoring and optimization.
Example AI Investment Calculation
- AI software cost: $500K per year
- Implementation & training: $100K (one-time cost)
- Ongoing AI maintenance: $50K per year
Total AI investment in year one:

Total AI investment in subsequent years:
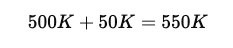
AI’s cost structure must be transparent and scalable. If AI adoption requires ongoing costs that outweigh its benefits, it will struggle to gain long-term executive support.
Step 5: Final AI ROI Calculation
With revenue gains, cost savings, and AI investment costs clearly defined, the final step is to calculate the overall return on investment.
Using the ROI formula:

Applying this to our earlier numbers:
- Revenue Gains: $2M
- Cost Savings: $1.3M
- AI Investment: $650K
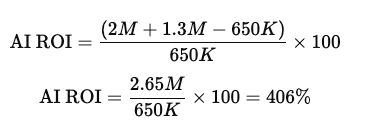
Interpreting the Results
In this example, AI delivers a 406% return on investment—a compelling business case for AI adoption.
Executives don’t invest in AI for automation alone—they invest when AI’s financial return is clear. This structured approach ensures CMOs present a quantified, data-driven argument for AI investment, rather than relying on AI’s broader industry momentum.
AI ROI Must Be a Measurable Business Metric
AI in marketing isn’t a cost—it’s an asset that must be evaluated like any other business investment. By following this framework, CMOs can ensure that AI initiatives are:
- Aligned with business objectives and financial targets.
- Connected to measurable revenue impact and cost savings.
- Justified with a clear ROI calculation to secure executive buy-in.
The next step? Building a financial model that projects AI’s long-term impact on marketing performance and scalability.
Making the Case: How to Get Executive Buy-In
Calculating AI’s return on investment is one thing—getting the CFO, CEO, and Board to approve the investment is another. While CMOs see AI as a transformative force in marketing, most executives aren’t interested in the technology itself. They care about business outcomes—growth, efficiency, and profitability.
No matter how advanced AI becomes, its success in the organization depends on one key factor: whether leadership sees it as a strategic, financially sound investment. That means the way AI is presented matters as much as the numbers behind it.
Framing AI for the Executive Audience
Executives are not looking for AI adoption for the sake of innovation. They are evaluating AI as a business decision, just as they would with any other investment in technology, personnel, or operations. The most successful AI proposals focus on how AI aligns with core business priorities.
When presenting AI to leadership, CMOs must answer these critical questions:
- How will AI drive revenue growth?
- How will AI reduce costs and improve efficiency?
- What is the financial return on AI compared to other strategic investments?
- What are the risks, and how can they be mitigated?
- How will AI adoption impact the competitive position of the company?
By grounding AI discussions in measurable business impact, CMOs shift the conversation from "we need AI" to "AI will create X% in revenue growth and Y% in cost savings."
Key Strategies for Securing AI Investment
1. Tie AI Directly to Business Goals
Executives don’t invest in AI—they invest in growth, profitability, and competitive advantage. If AI can’t be connected to a broader business objective, it will be viewed as an unnecessary expense rather than a strategic priority.
When making the case for AI, frame it around tangible business goals:
- Revenue Growth: AI-powered personalization can increase conversion rates by 15%, translating to millions in additional annual sales.
- Operational Efficiency: AI-driven automation can reduce manual workload by 30%, allowing teams to execute more campaigns without increasing headcount.
- Customer Retention: AI-driven churn prediction can improve customer retention by 10%, preserving millions in recurring revenue.
The more AI aligns with C-suite priorities, the stronger the business case becomes.
Example: Instead of saying, “AI will optimize our campaign performance,” say:
“AI-driven campaign optimization is projected to improve marketing ROI by 20%, increasing quarterly revenue by $5M.”
2. Show a Clear ROI Projection—Not AI Hype
The CFO doesn’t approve investments based on industry trends or vendor promises. AI proposals must be backed by clear financial projections, showing exactly how AI contributes to revenue and cost efficiency.
Instead of saying, “AI will improve customer insights,” provide hard numbers:
- Projected Revenue Impact: AI-powered lead scoring will increase win rates by 10%, resulting in $3M in additional revenue.
- Cost Savings: AI-driven media buying will cut wasted ad spend by 20%, saving $1M annually.
- Efficiency Gains: AI automation will eliminate 1,500 hours of manual work per year, reducing labor costs by $500K.
The best way to eliminate skepticism is to present data-driven projections that align with financial and operational goals.
3. Start with a Low-Risk AI Pilot Before Scaling
Executives are unlikely to approve a full-scale AI transformation overnight. A phased approach—starting with a targeted AI initiative—reduces perceived risk and demonstrates early success.
How to structure an AI pilot:
- Choose a focused, high-impact use case: AI-driven lead scoring, AI-powered ad optimization, or AI-based personalization.
- Define a clear success metric: If AI can improve conversion rates by 10% in a pilot, that validates the case for broader adoption.
- Show results quickly: A 3-6 month pilot allows leadership to see early ROI before committing to enterprise-wide AI adoption.
A small, measurable win builds credibility and turns AI from a theoretical investment into a proven business accelerator.
4. Frame AI as a Competitive Advantage—Not Just a Marketing Enhancement
Executives don’t just want better marketing—they want market leadership. The most compelling AI proposals position AI as a way to outperform competitors, not just optimize internal processes.
AI adoption should be framed as a strategic advantage that future-proofs the business. Without it, the company risks falling behind competitors who are already leveraging AI to scale faster, personalize customer experiences, and make smarter decisions.
The AI conversation should be as much about preventing competitive risk as it is about improving marketing execution.
CMOs Who Can’t Prove ROI Won’t Get AI Budgets
AI in marketing isn’t a trend—it’s a business decision. No matter how advanced AI becomes or how transformative its potential, investment won’t happen unless CMOs can clearly articulate the financial return.
AI doesn’t sell itself. The promise of automation, efficiency, and personalization means little without a direct connection to measurable business impact. Executives aren’t investing in AI because it’s innovative; they’re investing because it can increase revenue, reduce costs, and improve marketing performance at scale.
The CMOs who win AI budgets aren’t just the ones who understand AI—they’re the ones who can prove AI’s value in financial terms. They frame AI investments in revenue impact, cost efficiency, and competitive advantage. They present AI as a business accelerator, not a technical upgrade.
The reality is simple: CMOs who can quantify AI’s ROI will secure investment and drive meaningful AI adoption. Those who can’t will be left explaining why AI hasn’t delivered.